The Saskatchewan government hopes two technologies will help reduce solid waste dumped at landfills across the province.
Winners from the latest Innovation Challenge by Innovation Saskatchewan have created their own systems that aim to give the province a better idea of how much solid waste is entering waste facilities, and where they go.
Prairie Robotics Inc. and researchers from the University of Regina presented their respective solutions to stakeholders and dignitaries at the University of Regina on Tuesday afternoon.
As part of their success with the challenge, each group won $10,000 in funding and a 16-week residency to develop their projects while working with the government.
Kelvin Ng, an environmental systems engineering professor at the U of R, and his team designed a low-cost weight sensor unit called the LWsensor. It’s purpose is to measure the weight of the vehicle before and after entering a landfill or waste facility.
During his presentation, Ng mentioned they faced some challenges when creating the unit – most notably finding a way to make a product that can withstand the province’s harsh winter weather. He said their solution for this problem was to use heat cables in order to keep the temperature of the sensors constantly above O°C.
Ng feels it’s important to have an accurate estimate on solid waste data since research shows Canadians produce more solid waste than other countries.
“This is especially important for us in Saskatchewan because we have lots of empty space, which is suitable for landfill technologies,” said Ng. “It’s important for the researcher to develop so that we can have more effective and efficient waste management systems.”
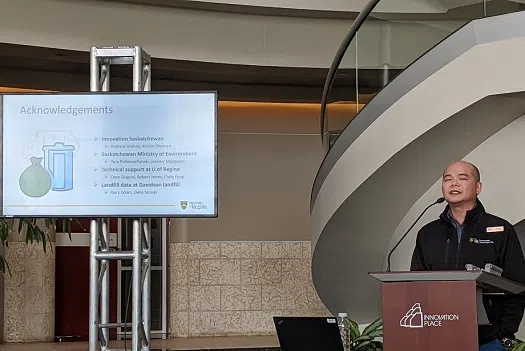
Kelvin Ng describing his team’s work to the audience on Tuesday at the University of Regina’s Innovation Place. (Photo: Moises Canales/620 CKRM)
The next step for Ng and his colleagues is to work with industrial partners to build a working prototype and eventually install the sensors at waste facilities in Saskatchewan. He believes there is a huge market for them to present low-cost systems to estimate the total amount of waste in Canada.
Sam Dietrich, a co-founder with Prairie Robotics, explained that his team’s system uses visual images from cameras on landfill sites and machine learning to estimate the amount and type of waste dropped off at each landfill in real time and create reports.
The team at Prairie Robotics also had their share of challenges they had to overcome during the development process. One answer they found to prove their system could produce good data was by partnering with the City of Regina to access four years of archival data.
As Dietrich put it, one of their team members hand-scrubbed those archival images, meaning they went through tens of thousands of images to manually verify their findings.
He said their unit can help municipalities across Saskatchewan better understand how much solid waste is being disposed of by their residents.
“If you don’t have a weigh scale and you can’t properly understand the amount of waste you’re receiving, it’s difficult to develop and design recycling and diversion programs,”suggested Dietrich.
Kari Harvey, CEO of Innovation Saskatchewan, said the groups will now work with the Ministry of Environment to look at developing their ideas in the province.
“We don’t expect it to be at a place where it can be commercialized, but to a place where we can see if it’s a technology that can be further developed and at some point be commercialized.”
Last month, the provincial government introduced their Solid Waste Management Strategy which unveiled that every Saskatchewan resident produces an average of 842 kilograms of garbage per year – the second-most per capita among the provinces.